How to use data warehouse for data analysis?
Before diving into our article, let’s take a look at the data warehouse! A data warehouse is a subject-oriented, integrated, relatively stable data collection that reflects historical changes.
Let’s look at these words:
- Topic-oriented. The data warehouse will plan various business topics, so we need to understand the scope of the major topics and the relationship between them, so that we can understand the basic structure of the data warehouse.
- Integrated. The data of the data warehouse will come from various business system data or external crawling data, so we need to know which source the model fields of each data warehouse come from, so that we can quickly and comprehensively understand the relevant business.
- Relatively stable. The data in the data warehouse generally does not change in real time, so we look at the data of last year today and look at the data of last year tomorrow. If we find that a certain monthly data is wrong, we may need to re-aggregate the daily data of the historical month.
- Reflect historical changes, which is why forecasting generally requires data analysts to shine.
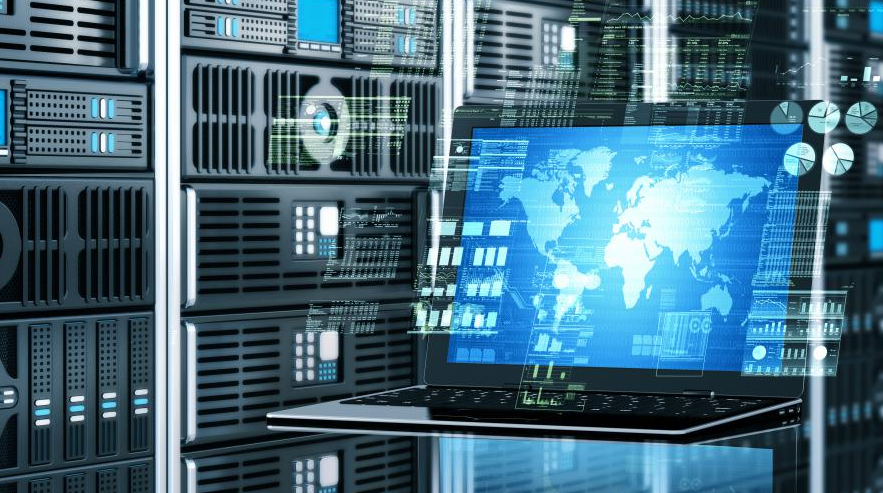
How to use data warehouse to optimize data analysis?
First of all, what is data analysis? Based on business requirements, combined with historical data, relevant statistical methods and some data mining tools and algorithms are used to integrate and analyze data, and form a set of solutions to finally solve a business scenario.
I heard from the teammates that in the process of data analysis, most of the work is dealing with data (most of which I think is 60% of the workload), so in order to improve work efficiency and quality, data analysis with the help of data warehouse is a great option.
How to use the data warehouse?
- To understand the original data. To truly understand the indicators, you must understand the original detailed data, know where it came from, and what dimensions were calculated.
- Look for “clean” data. Data analysis requires that the data be “clean” (can be input as algorithmic features), and the models in the data warehouse generally meet your requirements. We need to find a “clean” model, but the truth is often not very smooth, we need to find similar data, and then find the same link (association condition) to summarize the data by ourselves.
- Feedback data. After the data analysis has completed the analysis plan, it can share the results with the data warehouse partners, so that the data warehouse colleagues can learn the data analysis ideas, and can also better plan the model, thus entering a virtuous circle.
The organizational structure of both data warehouse and data analysis exists in many large teams, and many small teams do not have dedicated data analysts or data warehouse personnel, and the two are integrated.
Conclusion
Thank you for reading our article and we hope you’ve enjoyed it. If you want to learn more about data governance, we would like to advise you to visit Gudu SQLFlow for more information.
As one of the best data lineage tools available on the market today, Gudu SQLFlow can not only analyze SQL script files, obtain data lineage, and perform visual display, but also allow users to provide data lineage in CSV format and perform visual display.